What makes a good AI for insights?
In this blog, the first in a series created with Market Logic’s AI-for-insights experts, we put into plain English what you need to know about gen-AI for business, so you can avoid wasting time on AI for insights tools that won’t hold water when it comes to enterprise knowledge and insights acquisition.
This knowledge is essential for organizations today, who face a unique challenge: you’re in a race against your competitors to integrate generative AI (gen AI) into your business, but you can’t take risks that come with the rapidly developing technology, including investing too much time and resources on solutions that don’t fit your enterprise reality.
What you need to know about AI insights approaches today
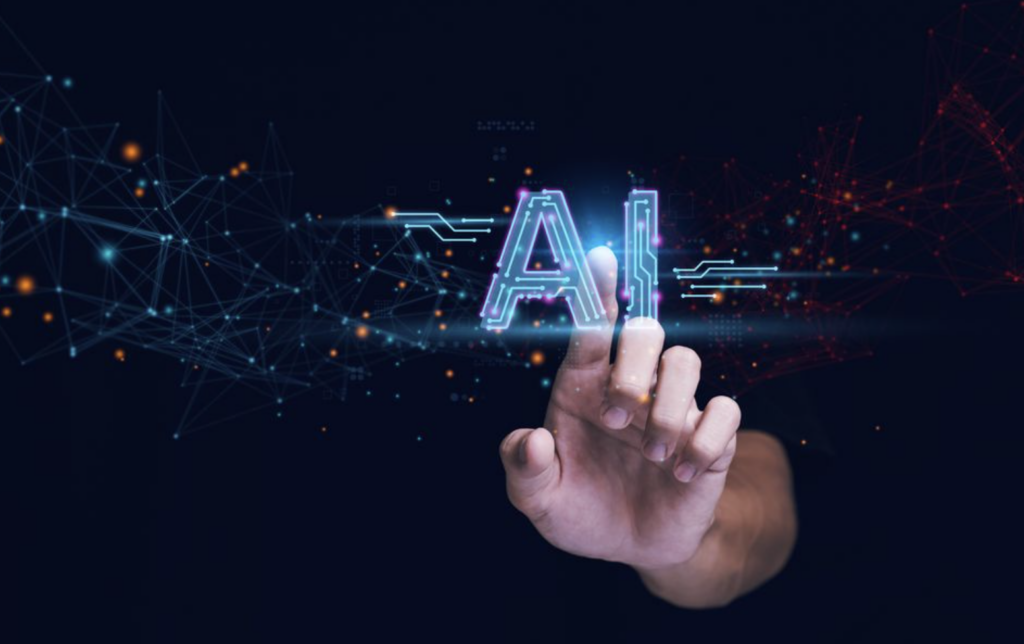
To understand what makes a good AI and a not-so-good AI for enterprise market insights, you need to know a bit about today’s go-to approach for leveraging the power of Large Language Models (LLMs) alongside your organization’s proprietary data.
Today’s go-to approach for infusing an enterprise’s private knowledge into a gen-AI model is called Retrieval-Augmented Generation (RAG).
If your eyes glazed over after seeing the words “retrieval-augmented generation,” fear not. Here’s something more digestible — figuratively, and literally speaking.
Creating a gourmet meal: an analogy for RAG-based gen AI tools
Think of a RAG-based gen AI tool as facilitating an overall process that starts with an input (a question or a prompt) and ends with an output (an answer).
Within this process is an architecture of algorithms, retrieval mechanisms, and LLMs, all of which work together to retrieve the best information from your organization’s proprietary knowledge to answer your question.
Creating a gourmet meal — an analogy for RAG — in five steps
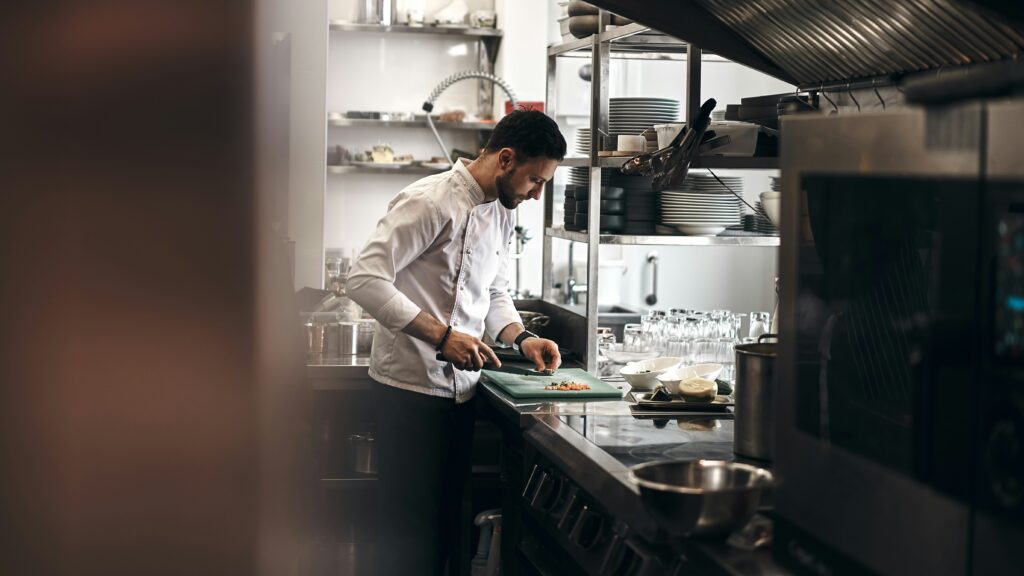
- User input: You can liken user input (i.e., a question or prompt) in a RAG-based AI tool to a customer at a restaurant specifying what they want to eat, possibly with certain dietary restrictions or flavor preferences. Just as a chef uses the customer’s request to guide the creation of a meal, a gen-AI solution uses a business user’s question to generate a relevant response.
After you ask your question, there’s a whole process kicked off in the background before you get your answer. To help you visualize that process, we’ve broken it down into three components. Rather than a step-by-step process, these components are more like complementary parts of an iterative, overlapping process — working synergistically towards the AI output.
2. Retrieval: You’ve ordered your meal (i.e., you’ve asked your question) and now the AI needs to retrieve an answer for you. Think of the retrieval component in a RAG-based AI tool as the chef unlocking their private pantry (i.e., your organization’s proprietary knowledge assets) to find the right ingredients (i.e., relevant pieces of information) for the dish. The pantry is extensive but manageable, with an array of spices, vegetables, meats, and other essentials. The chef must choose the right ingredients because it’s fundamental to ensuring the final dish (i.e., the AI’s output) matches the customer’s meal request.
3. Generation: The chef starts cooking, combining the ingredients they chose from their pantry in a specific way to create a delicious meal. The chef’s skills and experience are akin to an LLM’s broad training on massive amounts of general documents. Their training allows them to know how to mix the ingredients, how long to cook them, and what techniques to use to bring out the best flavors. This is akin to how an LLM’s training on large amounts of general-purpose information allows it to understand how to write like a human.
4. Integrating retrieved information: This component occurs within the generation phase. This is where, with the customer’s request in mind, the chef tastes the dish as it comes together, adjusting the seasoning, or perhaps adding a pinch of something that was missing. The chef needs to integrate the flavors in a way that ensures the dish is balanced, delicious, and, most importantly, expertly meets the customer’s request. In the RAG context, this step ensures that the information pulled from the database blends seamlessly with the generated content—making the final output not just accurate but also perfectly tailored to your internal knowledge-based query.
Real-life business alert: If the RAG solution is not fine-tuned and calibrated to your enterprise context in steps 2-4, you can end up with an output that’s imprecise or altogether off. Keep reading to learn what to look out for.
5. AI output: The final dish served to the customer, beautifully plated and ready to eat, represents the AI’s output. It’s the culmination of the chef’s work, prepared using the right ingredients, cooking techniques, and presentation skills. In the RAG context, AI output is the final answer or content delivered to the user, crafted to be informative, accurate, and trustworthy.
The difference between a generic AI solution and a robust, purpose-built gen-AI solution for your enterprise reality
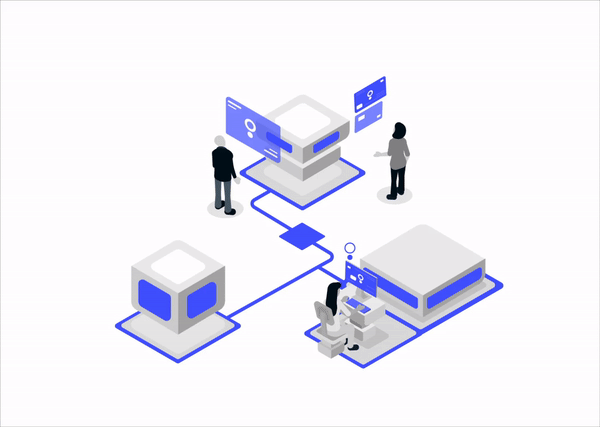
The analogy above is a “blue-sky” scenario for how a RAG-based AI tool works. The problem is that the analogy gets a little tricky when it’s put into the context of today’s enterprise insights functions.
Today’s enterprises have a lot of knowledge. Most of the organizations we work with, for example, have hundreds of thousands of proprietary knowledge assets, including research reports, market analyses, customer feedback, tests, reviews, news, syndicated sources, and much more. Usually, standard RAG-based gen-AI tools run into problems when faced with the large amounts of information common to most enterprises.
Using our analogy above, here’s how that problem plays out for the chef:
When the chef goes into their exclusive pantry to retrieve ingredients for the meal, instead of a manageable yet extensive pantry of ingredients, they walk into a warehouse with millions of ingredients.
They are overwhelmed by the number of options available, but as they want to make the best dish, they must look at everything that could possibly be relevant to the customer’s request.
For example, the customer asked for a gluten-free pizza. In this case, the chef automatically retrieves every gluten-free ingredient in the warehouse, even though most of the ingredients are only somewhat related and essential for the core flavors of a pizza.
As there isn’t enough time to sort through every ingredient brought back from the pantry to the kitchen, the chef ends up picking the ingredients closest to them, out of convenience and even if they’re not right. As a result, the final dish (i.e., the answer to your question) ends up being a confusing mix of flavors, or rather, flavors that are irrelevant to the customer’s order.
In the RAG gen-AI tool context, this problem could look like this example adapted from Market Logic’s Co-Founder, Chief Innovation & Product Officer Olaf Lenzmann in his recent article explaining how Market Logic has experimented with optimizing our evidence classification in the RAG process:
A marketer asks: How does Gen Z in Spain feel about climate change?
The AI tool answers: 68% of Gen Z respondents in Spain report being concerned about social justice and its lasting effects on society.
But there was a better answer hidden in the background: 88% of respondents are somewhat or very pessimistic about the effects of global warming. (n=245, m/f, age 18–24, Madrid & Barcelona)
To summarize, just because the ingredients are the most “convenient” it doesn’t necessarily make them the most relevant. This applies to your RAG.
Conclusion: A good generative AI is tuned to the real-world enterprise context
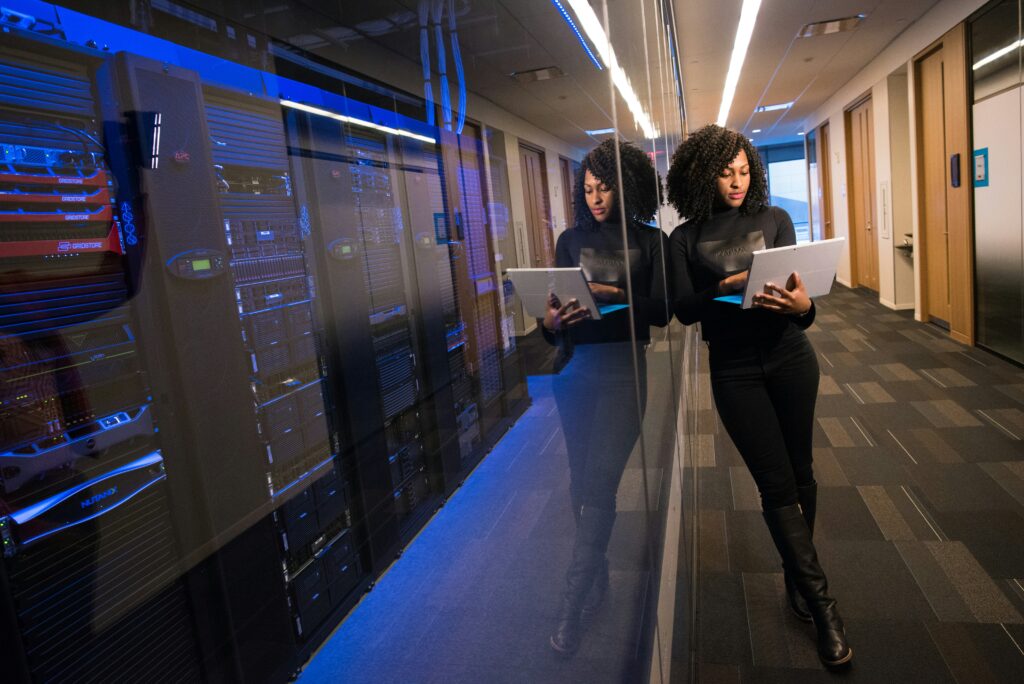
Most of the enterprise/business generative AI tools you’re experimenting with today are RAG-based. But the difference between a cool demo from a vendor and a robust real-world business application is in the details of how the tool is refined to be effective in the enterprise context today and in the future.
To recap:
- A generic RAG gen AI tool can fail to answer business questions with truly relevant content because it’s been drowned out by the sheer volume of tangentially related information.
- Without a refined method of discerning which pieces of information are truly relevant, it can lead to a final output that, while somewhat on-topic, may miss the mark in directly addressing a business user’s specific question.
- Just as the chef needs to be discerning in ingredient selection to maintain the integrity of the dish, the RAG system requires a more sophisticated retrieval strategy to ensure that only the most relevant information is passed on to the generation component, preserving the precision and relevance of the AI’s output.
In our next blog post, you’ll learn how the gen-AI tool DeepSightsTM is refined and calibrated, so you get relevant, reliable answers to your business questions.
We’ll continue the analogy with the chef and learn how — with the right domain knowledge and context — they avoid the problem of being overwhelmed by a warehouse of ingredients (i.e., how your AI solution ensures that only the relevant context is picked).
Try generative AI for enterprise insights
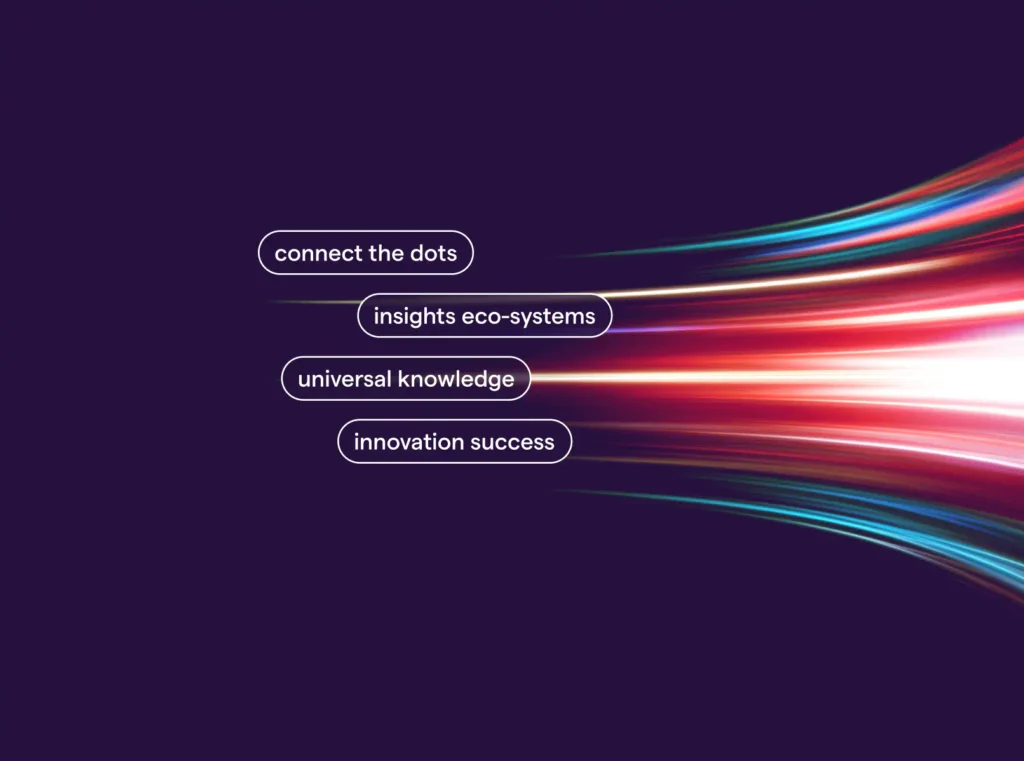
A good RAG-based AI tool is refined and calibrated from its inception so that you get relevant, reliable answers to your business questions. Market Logic takes the real-world context of enterprise insights seriously and then builds that enterprise-grade quality into their generative AI tool DeepSightsTM — so you don’t have to worry about scaling instant, reliable insights across your organization.
Do you want trustworthy, relevant answers from your knowledge base within seconds? Check out DeepSightsTM, the first AI assistant for market insights, including a one-click report generator.