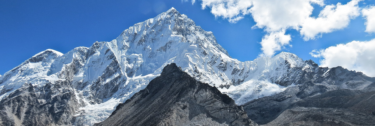
November 30, 2016
Read time: 3min
November 30, 2016
Read time: 3min
In one of a series of essays on the impact of AI on the insights profession, our cognitive guru identifies a key tactic to help marketers find the insights they need efficiently from an ever-growing mountain of consumer data. The technology on the table: pre-defined marketing relationships in your AI knowledge graph.
Have you ever noticed that a Google search for “Mount Everest” results in a wiki summary displaying elevation and first ascent, but a search for “Beyoncé” displays the name of her children and her date of birth? You’re looking at a knowledge graph that is automatically generated by cognitive computing algorithms. Inside a Google search, knowledge graphs deliver a wiki summary and synthesized facts from the most frequent results for your query, derived from all search results.
While the ability to automatically summarize results in a dynamic knowledge graph is very helpful for general searches, it’s not at all helpful when marketers are trying to make sense of all their research and data on an insights platform.
Let’s say you’re a brand manager for a shampoo product and you’re searching Google for hair care problems. You type in “dry, brittle hair”. Unlike your Mount Everest and Beyoncé queries, the results will not reveal a knowledge graph. That’s because the number of search results about the specific attributes that could be used to describe dry, brittle hair are too broad to use to identify key characteristics. As a consequence, general cognitive computing approaches to knowledge graph development simply don’t deliver actionable results for marketers and researchers.
At Market Logic, we turn this situation around for our clients by training their insights platforms to think like marketers. How so? When a marketer contemplates an opportunity or problem, they synthesize information in a mental map that reflects basic marketing concepts – who is the consumer, what are their needs, and so on. We do the same by pre-defining relationships in the knowledge graph. For example, the knowledge graph knows that consumer segments have needs, which are fulfilled by brands, that offer RTBs. Because it understands these relationships, the machine can look for patterns to identify concepts (i.e. negative sentiment toward something about a brand could be a barrier). This pre-defined “market logic” can then learn and apply the language of a category – so the machine understands that “dryness” is a barrier to hair care and a benefit for diapers. Further, it can classify, cluster and detect patterns between concepts, so relationships between consumers and events, for example, could portend emerging trends.
Let’s say the shampoo brand manager works at Unilever. When she searches her PeopleWorld insights platform, powered by Market Logic, the machine understands what’s needed, searches the vast repository of research and social media for hair problems like hair loss and dandruff, and instantly delivers an actionable, high-level overview. The knowledge graph paints a clear picture of the distinct and overlapping hair care problems in a market. According to Harvard Business Review, that’s information that can yield insights about consumer needs and innovative opportunities to meet the market demands. No more needless Google searches.